Table Of Content
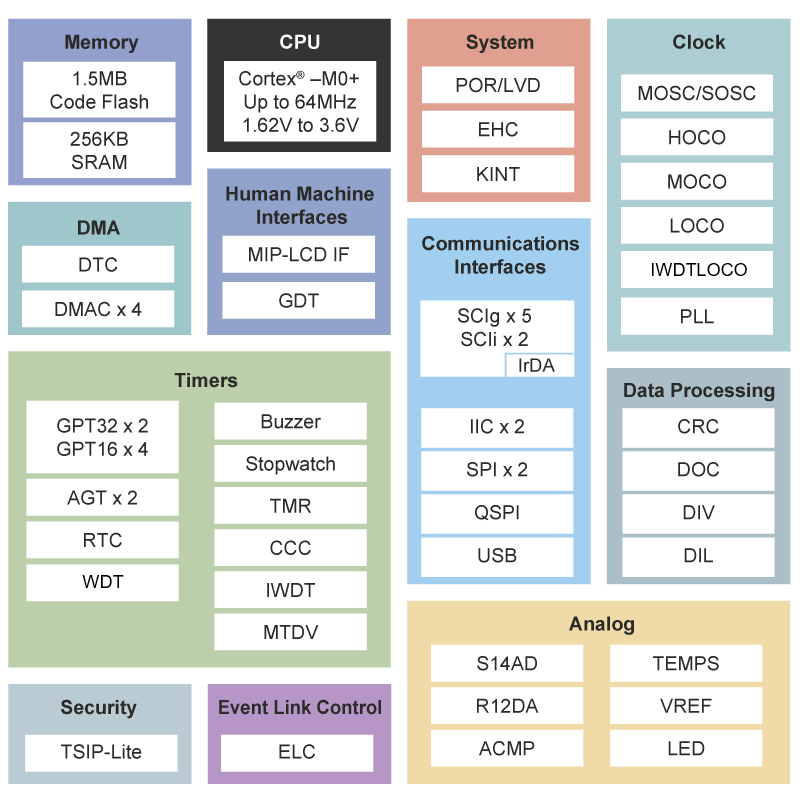
To do a crossover design, each subject receives each treatment at one time in some order. So, one of its benefits is that you can use each subject as its own control, either as a paired experiment or as a randomized block experiment, the subject serves as a block factor. The number of periods is the same as the number of treatments. It is just a question about what order you give the treatments.
Table of contents
When we have complete data the block effect and the column effects both drop out of the analysis since they are orthogonal. With missing data or IBDs that are not orthogonal, even BIBD where orthogonality does not exist, the analysis requires us to use GLM which codes the data like we did previously. If you look at how we have coded data here, we have another column called residual treatment. For the first six observations, we have just assigned this a value of 0 because there is no residual treatment. But for the first observation in the second row, we have labeled this with a value of one indicating that this was the treatment prior to the current treatment (treatment A). In this way the data is coded such that this column indicates the treatment given in the prior period for that cow.
Randomized Block Design
We will achieve a smaller P obtained value, and are more likely to reject the null hypothesis. In other words, good blocking variables decreases error, which increases statistical power. In our previous diet pills example, a blocking factor could be the sex of a patient. We could put individuals into one of two blocks (male or female). And within each of the two blocks, we can randomly assign the patients to either the diet pill (treatment) or placebo pill (control).
2 Split-plot designs
These plots provide more information about the constant variance assumption, and can reveal possible outliers. The plot of residuals versus order sometimes indicates a problem with the independence assumption. Suppose that there are a treatments (factor levels) and b blocks. In some disciplines, each block is called an experiment (because a copy of the entire experiment is in the block) but in statistics, we call the block to be a replicate. This is a matter of scientific jargon, the design and analysis of the study is an RCBD in both cases. Another way to think about this is that a complete replicate of the basic experiment is conducted in each block.
Assign treatments to blocks
More specifically, blocking is used when you have one or more key variables that you need to ensure are similarly distributed within your different treatment groups. The mathematical subject of block designs originated in the statistical framework of design of experiments. These designs were especially useful in applications of the technique of analysis of variance (ANOVA). In this case, we would have four rows, one for each of the four varieties of rice.
Interpretation of the coefficients of the corresponding models, residualanalysis, etc. is done “as usual.” The only difference is that we do not test theblock factor for statistical significance, but for efficiency. Unfortunately the above model isn’t correct because R isn’t smart enough to understand that the levels of plot and subplot are exact matches to the Variety and Fertilizer levels. As a result if I defined the model above, the degrees of freedom will be all wrong because there is too much nesting. So we have to be smart enough to recognize that plot and subplot are actually Variety and Fertilizer. One issue that makes this issue confusing for students is that most texts get lazy and don’t define the blocks, plots, and sub-plots when there are no replicates in a particular level.
Complete Block Designs
The fact that you are missing a point is reflected in the estimate of error. You do not have as many data points on that particular treatment. Before high-speed computing, data imputation was often done because the ANOVA computations are more readily done using a balanced design. There are times where imputation is still helpful but in the case of a two-way or multiway ANOVA we generally will use the General Linear Model (GLM) and use the full and reduced model approach to do the appropriate test. Basic residual plots indicate that normality, constant variance assumptions are satisfied. Therefore, there seems to be no obvious problems with randomization.
No Blocking Variable vs. Having a Blocking Variable
Above you have the least squares means that correspond exactly to the simple means from the earlier analysis. This website is using a security service to protect itself from online attacks. The action you just performed triggered the security solution. There are several actions that could trigger this block including submitting a certain word or phrase, a SQL command or malformed data.

The partitioning of the variation of the sum of squares and the corresponding partitioning of the degrees of freedom provides the basis for our orthogonal analysis of variance. The design is balanced having the effect that our usual estimators andsums of squares are “working.” In R, we would use the model formulay ~ Block1 + Block2 + Treat. We cannot fit a more complex model, includinginteraction effects, here because we do not have the corresponding replicates.
20+ Graphic Design Statistics That You Should Know in 2024 - G2
20+ Graphic Design Statistics That You Should Know in 2024.
Posted: Wed, 13 Mar 2024 07:00:00 GMT [source]
As a results, there will be three parts of the variance in randomized block ANOVA, SS intervention, SS block, and SS error, and together they make up SS total. In doing so, the error variance will be reduced since part of the error variance is now explained by the blocking variable. When the numerator (i.e., error) decreases, the calculated F is going to be larger.
Notice that if we only have one insertion per mouse, then the mouse effect will be confounded with materials. Where F stands for “Full” and R stands for “Reduced.” The numerator and denominator degrees of freedom for the F statistic is \(df_R - df_F\) and \(df_F\) , respectively. Plotted against block the sixth does raise ones eyebrow a bit.
Every binary matrix with constant row and column sums is the incidence matrix of a regular uniform block design. Also, each configuration has a corresponding biregular bipartite graph known as its incidence or Levi graph. Hence, a block is given by a locationand an experimental unit by a plot of land.
The nuisance factor they are concerned with is "furnace run" since it is known that each furnace run differs from the last and impacts many process parameters. It is impossible to use a complete design (all treatments in each block) in this example because there are 3 sunscreens to test, but only 2 hands on each person. A resolvable 2-design is a BIBD whose blocks can be partitioned into sets (called parallel classes), each of which forms a partition of the point set of the BIBD.
No comments:
Post a Comment